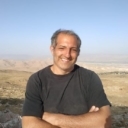
SafetyDetectives recently interviewed Mark Wright, the Director of Technical Marketing at GSI Technology, who brings over 25 years of experience in systems engineering. GSI’s flagship features, including their Gemini technology and APU implementation, revolutionize AI processing with high bandwidth, boolean processing, and single-bit programming granularity, enhancing efficiency and accuracy. Mark discusses the challenges of developing advanced memory solutions for AI applications and highlights GSI’s disruptive compute-in-memory architecture, poised to reshape AI processing efficiency, particularly for edge applications, with upcoming innovations promising 10x performance improvements and smaller footprints.
Could you introduce yourself and describe your role at GSI Technologies?
My name Mark Wright, and I’m the Director of Technical Marketing at GSI Technology. I have 25 years experience in systems engineering in high rel, industrial and commercial products in the embedded and networking space.
What are the flagship features of GSI Technologies, and how do they reflect the company’s vision?
GSI technology’s Gemini technology and APU implementation of the same uses SRAM technology to implement a compute-in-memory architecture that is very tightly coupled with a large SRAM working memory. This architecture provides flagship features of very high bandwidth from memory to compute structure, high speed boolean processing, single bit programming granularity, lower compute power and very high array utilization for certain AI inference and high performance compute workloads.
Can you explain how GSI’s “Disruptive Solution for Gen AI & Vector Search” is changing the landscape of artificial intelligence, particularly in terms of efficiency and accuracy?
This disruption comes in multiple ways. First the single bit granularity provides unrestricted software flexibility for both reduced weights and dynamic precision throughout the workflow. Without a restriction on framework definition, a user can chose to use 3 bit weights, or have a single “word” be 2000 bits wide to represent a molecule in a vector representation. Also, size of intermediate variables can be different lengths to address best accuracy without wasting resources. This provides efficiency of compute/memory resources. Efficiency is also provided by not having to move data from memory to compute structures as often as the computation is done in place, in memory.
What are the biggest challenges in developing advanced memory solutions for AI applications?
The biggest challenges in developing AI processing solutions are 1) you have a lot of data, and 2) getting that tremendous amount of data to the compute elements traditionally requires different levels of cache, each with higher bandwidth as you get close to the processing elements. This results in a behemoth problem of transferring data more than actually computing on it, and this results in massive expenditures of power for just data movement. The farther from the compute elements the data is the faster the memory solutions need to be in order to feed the system. Another problem that then comes up is 3) getting the results out without interfering with getting new data in. By making the core memory computation-capable, and then feeding it with a large, wide and efficient distributed register memory block, we have cleared the second and third problems and allow advancements everyone is using for memory solutions to address the large amount of data and throughput to have an even greater impact by using our architecture.
Where do you see the future of <SRAM> [compute-in-memory] technology, especially in AI contexts?
With the large amount of energy consumed in current AI processing just to move data around, we see more companies adopting compute-near-memory architectures for components and datacenter. With our unique solution that goes further and computes in memory, we see this as the enabling technology that could bring datacenter level performance at less SWAP (size, weight and power) for edge applications. This will open AI to many use cases where more effective and timely decisions can be made early in the data gathering or data transmission process.
Are there any upcoming projects or innovations at GSI Technologies that you can share with us?
Yes. We have shown the ability to do a number of AI inference processes in near real-time in a 1U server with our current production chip. These processes being SAR (synthetic aperture radar) image processing, body and face recognition, GSP denied navigation, and billion scale search. GSI has just taped out our second generation chip that brings 10x the performance in a smaller solution footprint. This innovation will reduce SWAP even further and open even more industrial AI and HPC applications at the edge. Also, we see this as such a paradigm shifting solution to the current power and throughput problems that exist in current AI solutions that we are welcoming discussions on IP licensing for other AI chip manufacturers to incorporate the technology even into small portions of their traditional solutions. We are particularly excited about this as we can show an order of magnitude improvement of LLM processing when this architecture is used. We see this as an enabler of “ChatGPT at the edge”.